Sitemap
A list of all the posts and pages found on the site. For you robots out there is an XML version available for digesting as well.
Pages
Page Not Found
Page not found. Your pixels are in another canvas.
About me
About me
Page not in menu
This is a page not in th emain menu
Posts
Best Mobile Manipulation paper finalist at IROS 2024
Our paper BaSeNet was among the 5 finalists for the best Mobile Manipulation paper award at the IEEE/RSJ Intelligent Conference on Robot and Intelligent Systems (IROS) 2024.
Successfully defended my PhD thesis
I successfully defended my PhD thesis titled “Pre-grasp planning for time-efficient and robust mobile manipulation” on 20 September 2024.
Paper accepted at IROS 2024
Our paper “BaSeNet “ has been accepted for publication in 2024 IEEE/RSJ International Conference on Intelligent Robots and Systems (IROS).
Paper accepted for publication in IEEE RAL
Our research on pre-grasp approaching on mobile robots has been accepted for publication in the IEEE Robotics and Automation Letters (RA-L) Journal.
Conclusion of the FacilityCobot Project
The FacilityCobot project has officially concluded. This project marked my initial foray into the field of Mobile Manipulation. My PhD research was mostly funded throught this project.
Visiting PhD student at METU
I spent January 2023 at Middle East Technical University (METU) in Ankara, Turkey, as a visiting PhD student. During this period, I collaborated with Prof. Sinan Kalkan to address the challenge of optimizing the time-efficiency of Mobile Manipulation.
Conclusion of the ReThiCare project
The ReThicare project has officially concluded.
Nordic Probabilistic AI Summer school
I attended Nordic Probabilistic AI Summer School in Helsinki, Finland.
Outstanding manipulation paper finalist at ICRA 2022
Our ICRA 2022 paper “Multi-view object pose distribution tracking for pre-grasp planning on mobile robots” is among the 3 finalists for the outstanding manipulation paper award.
Paper accepted at ICRA 2022
My very first submission related to my PhD research has been accepted IEEE International Conference on Robotics and Automation (ICRA) 2022
2 papers accepted at ROMAN 2021
2 of our papers related to interactive drink serving robot work, have been accepted at IEEE International Conference on Robot and Human Interactive Communication (ROMAN) 2021
I officially started my PhD
I officially started my PhD, at SDU Robotics, Faculty of Engineering, University of Southern Denmark.
Conclusion of the SMOOTH project
The SMOOTH project has officially concluded. This was my first-time experience working on interactive robots. Here is the video of our work on interactive drink serving robot which was one of the use case in the SMOOTH project.
I joined SDU Robotics
I moved to Odense, Denmark and joined the SDU Robotics group. To begin with, I will be working on the SMOOTH and RethiCare projects.
Conclusion of the ROPOD project
Today marks the official conclusion of the ROPOD project, representing the largest research endeavor I have been a part of to date.
I defended my master thesis
I successfully defended my master thesis titled “Semantic localization and navigation using OpenStreetMap”.
My first conference - ICRA 2019
I am in Montreal, Canada to attend my very first academic conference - ICRA 2019. I will be presenting our work on Semantic mapping extension for OpenStreetMap applied to indoor robot navigation
My first paper as a first author accepted at ICRA 2019
More details can be found here
publications
research
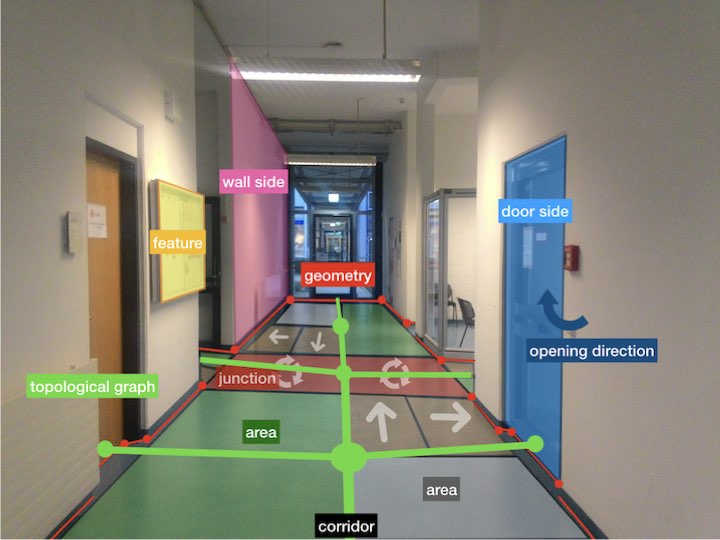
Semantic world modelling and indoor navigation using OpenStreetMap
We explored how digital navigation maps such as OpenStreetMap can be used for indoor robot navigation
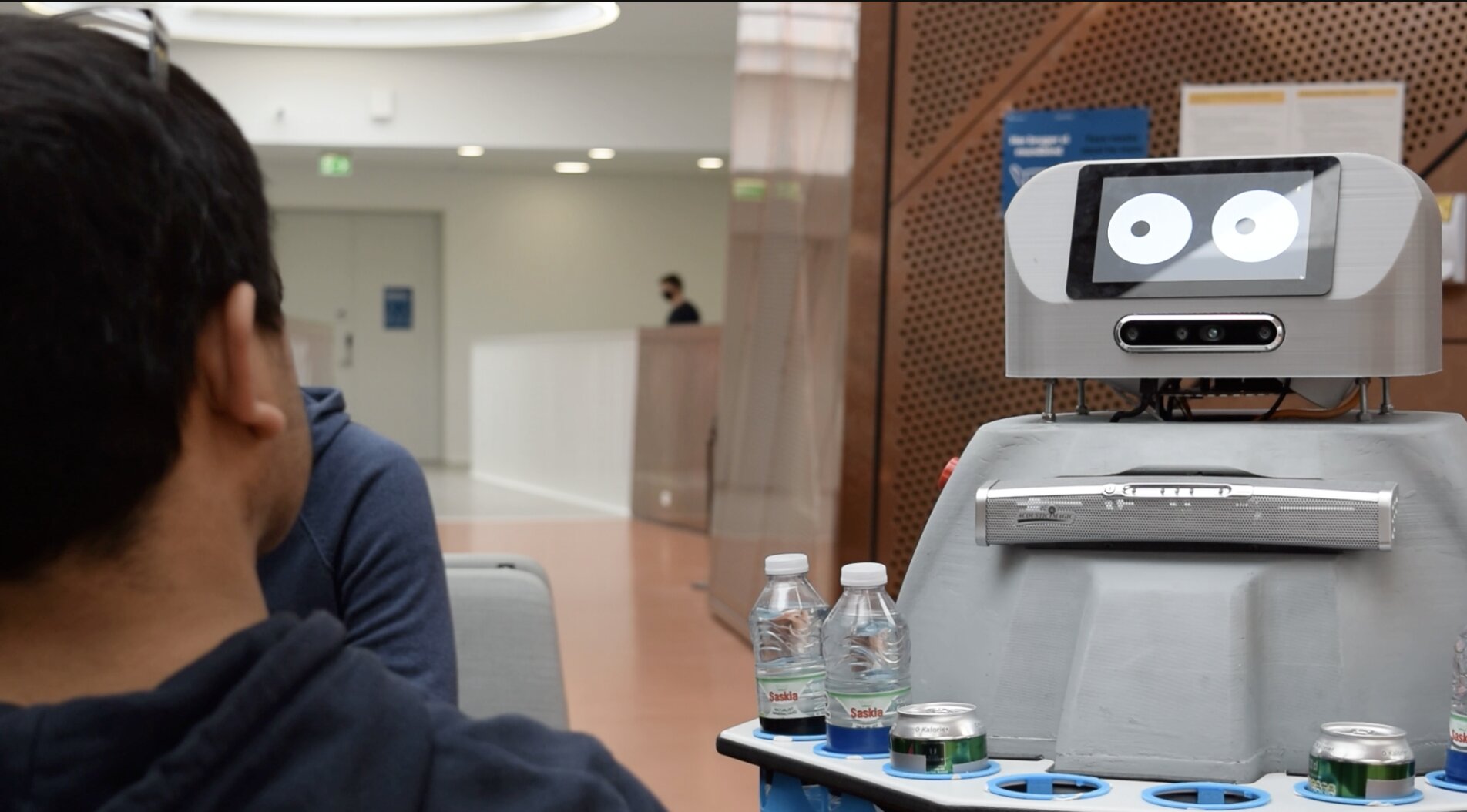
An interactive drink serving robot
We designed and implemented an interactive service robot capable of seamless deployment in real-world environments, coupled with high social acceptance.
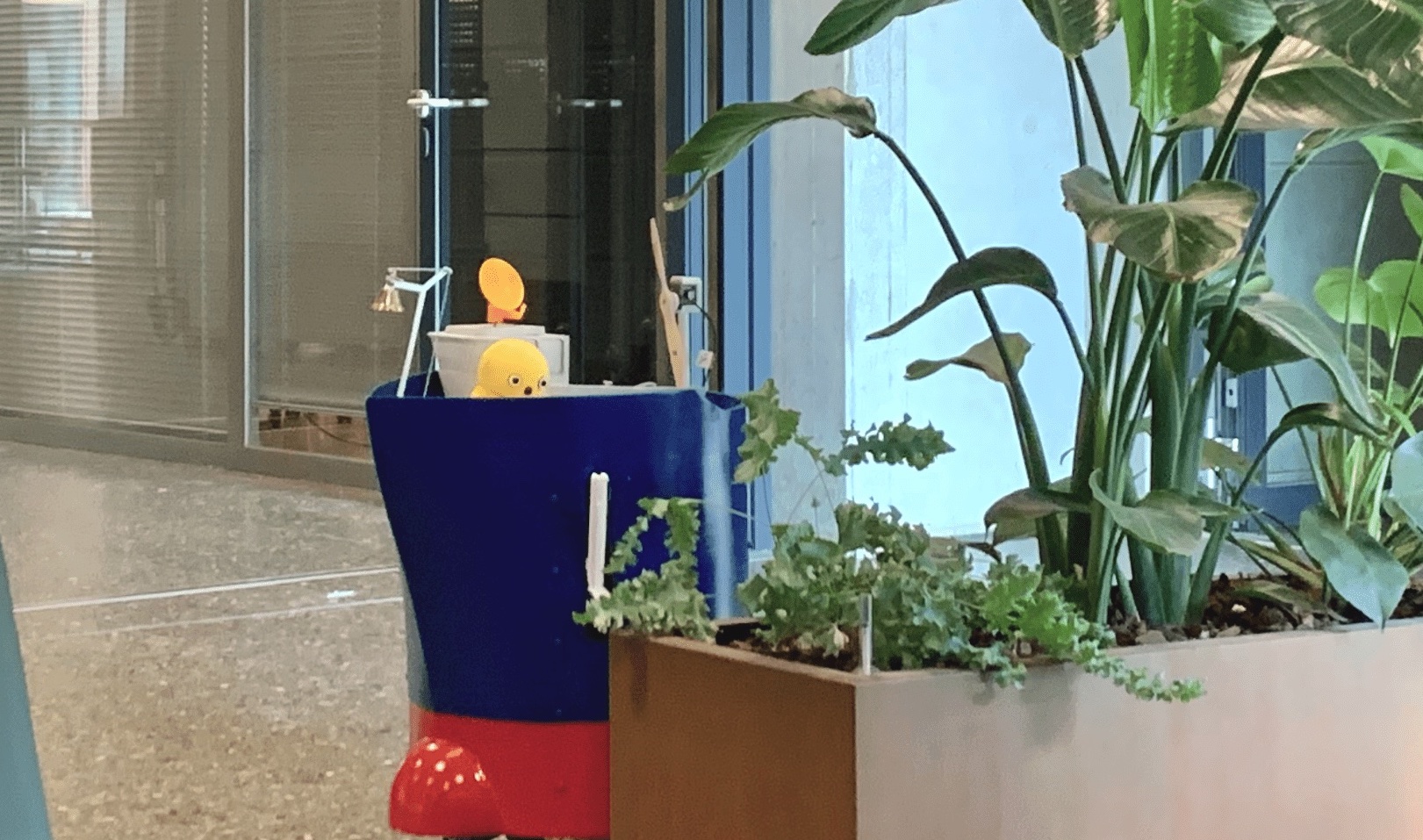
Distributed agency in HRI
We explored the use of distributed agency on mobile care robots using a prototype of the Plant Watering Robot
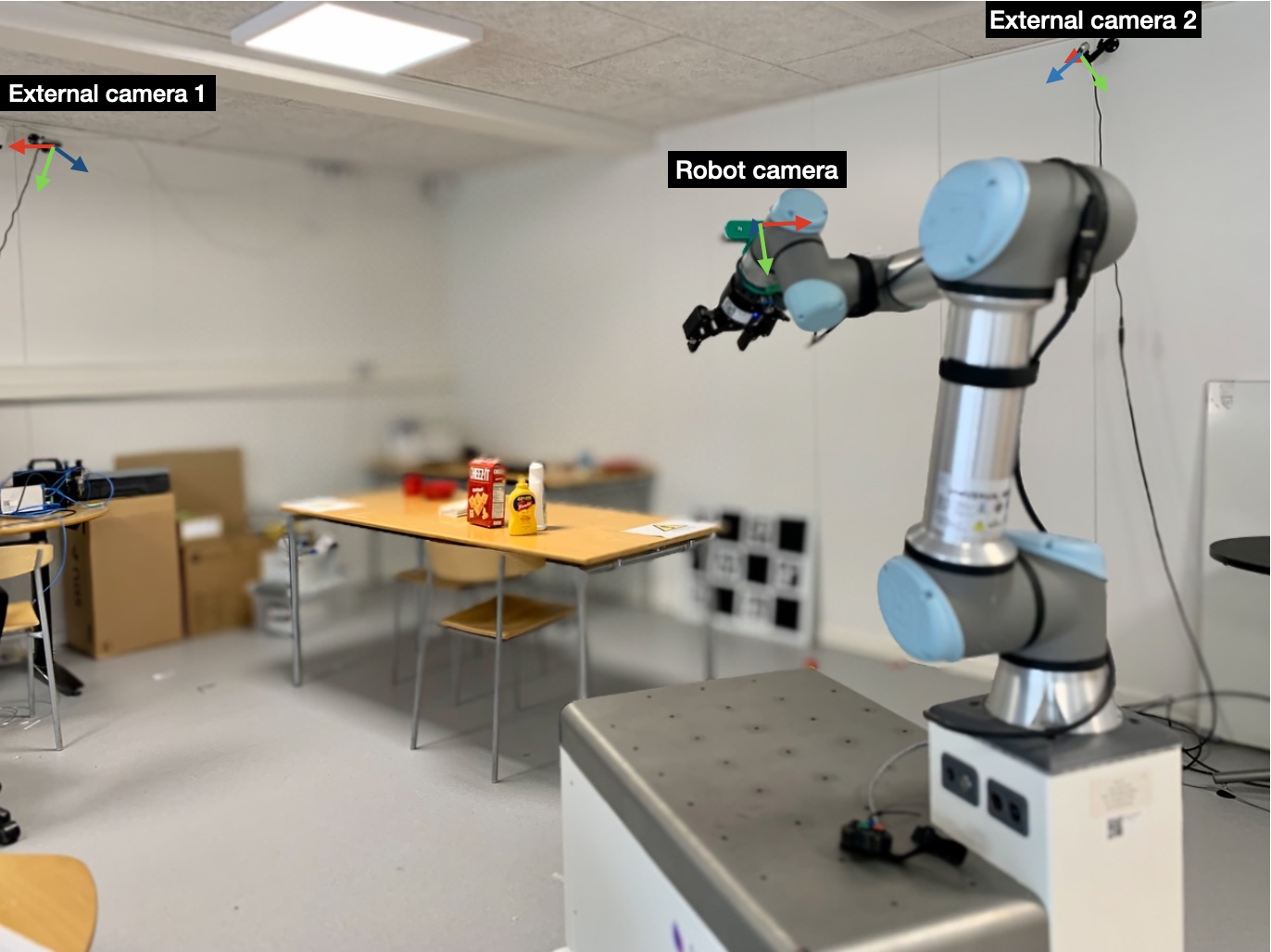
Multi-view object pose distribution tracking
We developed multi-view object pose distribution tracking framework for pre-grasp planniong on mobile robots
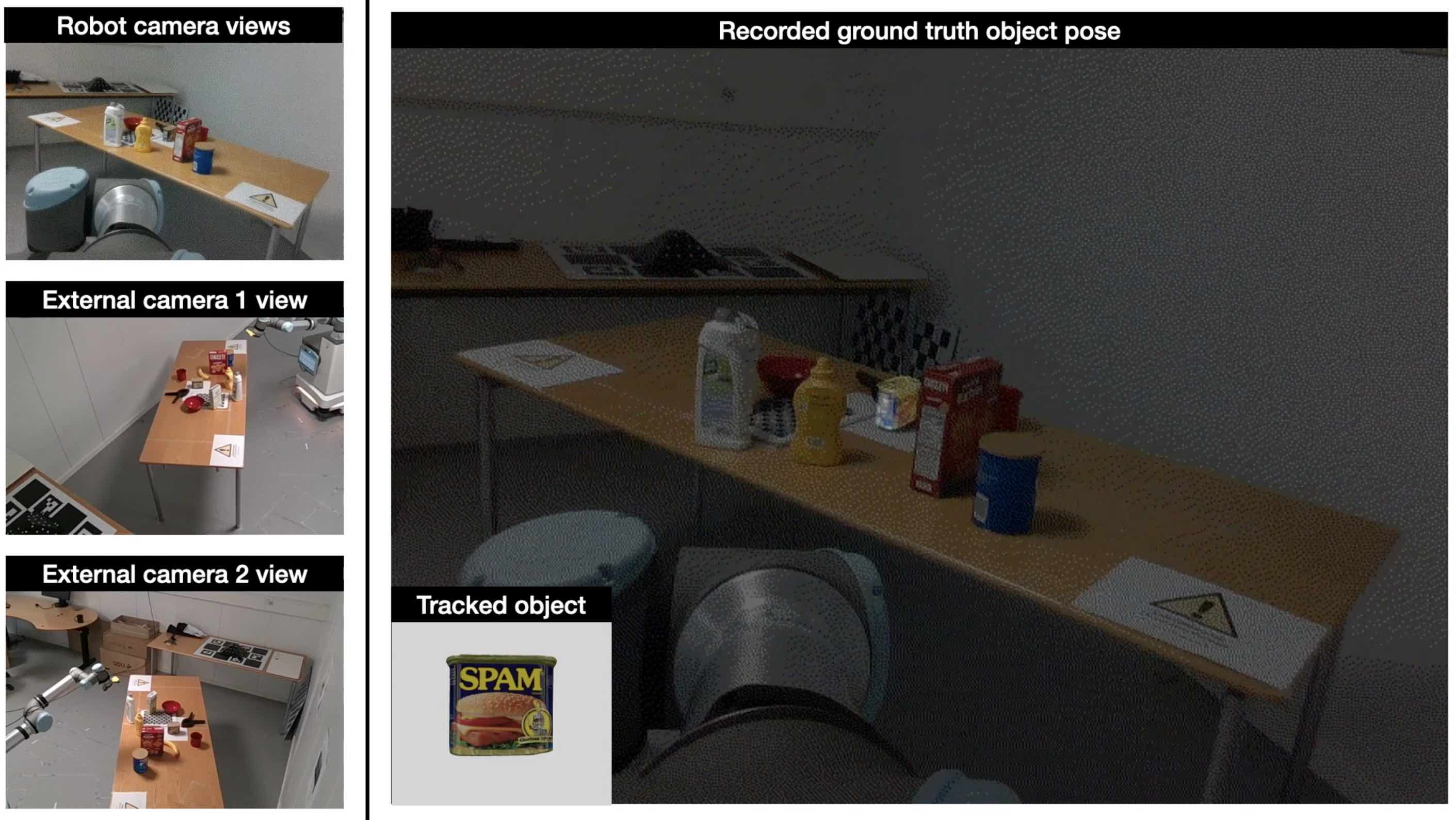
Multi-view YCB object pose tracking dataset for Mobile Manipulation
We released a Multi-view YCB object pose tracking dataset for Mobile Manipulation (MY-MM) with views of the objects from the robot's eye-in-hand and external cameras in the environment.
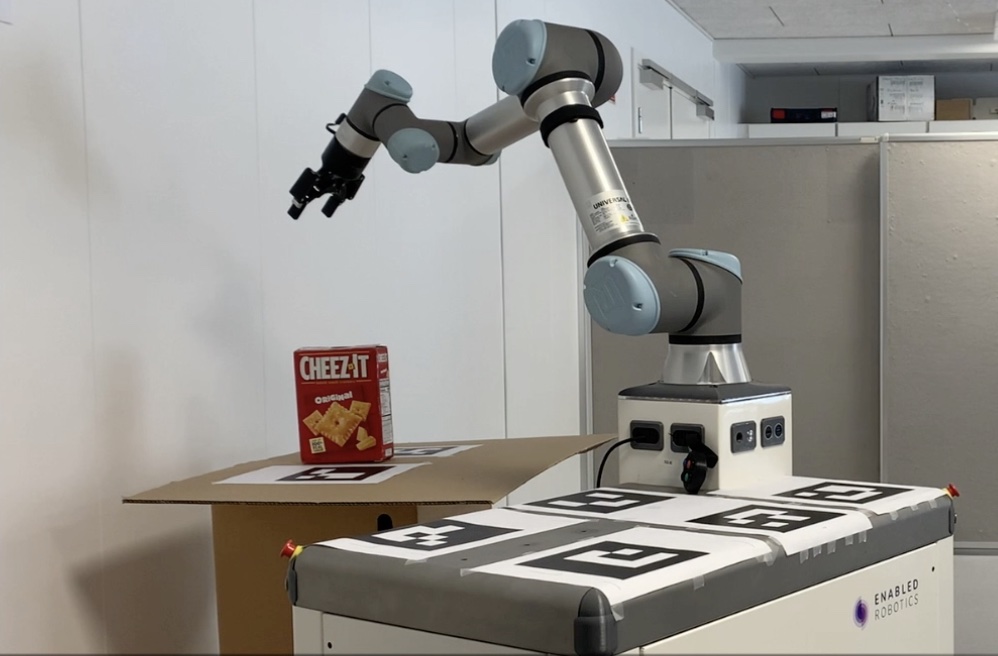
Pre-grasp approaching on mobile robots
In this research, we explored the use of a pre-active approach to determine a suitable base pose and pre-grasp manipulator configuration for grasping on mobile robots.
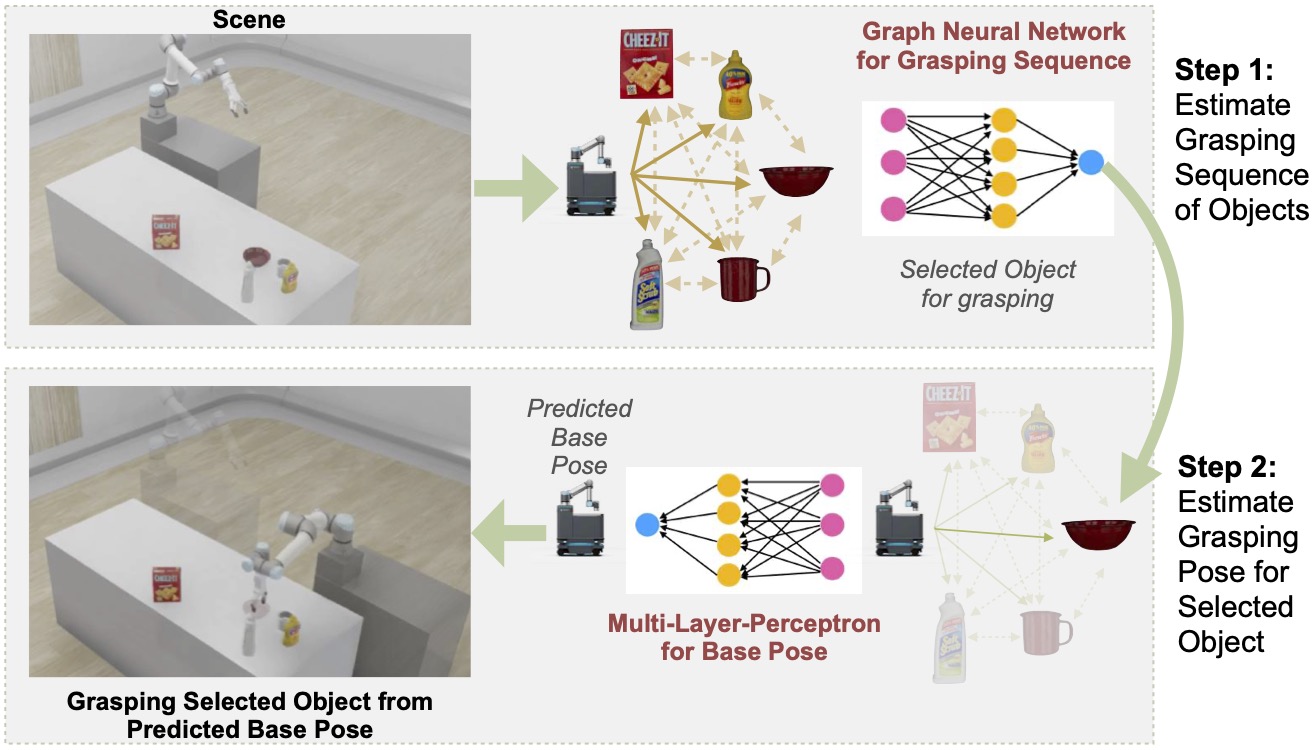
BaSeNET: A Learning-based Mobile Manipulator Base Pose Sequence Planning for Pickup Tasks
We present BaSeNET: a learning-based approach to plan the sequence of base poses for grasping objects on mobile robots.
talks
Semantic mapping extension for OpenStreetMap applied to indoor robot navigation
Abstract: In this work a graph-based, semantic mapping approach for indoor robotics applications is presented, which is extending OpenStreetMap (OSM) with robotic-specific, semantic, topological, and geometrical information. Models are introduced for basic indoor structures such as walls, doors, corridors, elevators, etc. The architectural principles support composition with additional domain and application-specific knowledge. As an example, a model for an area is introduced, and it is explained how this can be used in navigation. A key advantage of the proposed graph-based map representation is that it allows exploiting the hierarchical structure of the graphs. Finally, the compatibility of the approach with existing, grid-based motion planning algorithms is shown.
Multi-modal Proactive Approaching of Humans for Human-Robot Cooperative Tasks
Abstract: In this paper, we present a method for proactive approaching of humans for human-robot cooperative tasks such as a robot serving beverages to people. The proposed method can deal robustly with the uncertainties in the robot’s perception while also ensuring socially acceptable behavior. We use multiple modalities in the form of the robot’s motion, body orientation, speech and gaze to proactively approach humans. Further, we present a behavior tree based control architecture to efficiently integrate these different modalities. The proposed method was successfully integrated and tested on a beverage serving robot. We present the findings of our experiments and discuss possible extensions to address limitations.
Multi-view object pose distribution tracking for pre-grasp planning on mobile robots
Abstract: The ability to track the 6D pose distribution of an object when a mobile manipulator robot is still approaching the object can enable the robot to pre-plan grasps that combine base and arm motion. However, tracking a 6D object pose distribution from a distance can be challenging due to the limited view of the robot camera. In this work, we present a framework that fuses observations from external stationary cameras with a moving robot camera and sequentially tracks it in time to enable 6D object pose distribution tracking from a distance. We model the object pose posterior as a multi-modal distribution which results in a better performance against uncertainties introduced by large camera-object distance, occlusions and object geometry.
Multi-view object pose distribution tracking for pre-grasp planning on mobile robots
Abstract: The ability to track the 6D pose distribution of an object when a mobile manipulator robot is still approaching the object can enable the robot to pre-plan grasps that combine base and arm motion. However, tracking a 6D object pose distribution from a distance can be challenging due to the limited view of the robot camera. In this work, we present a framework that fuses observations from external stationary cameras with a moving robot camera and sequentially tracks it in time to enable 6D object pose distribution tracking from a distance. We model the object pose posterior as a multi-modal distribution which results in a better performance against uncertainties introduced by large camera-object distance, occlusions and object geometry.
Multi-view object pose distribution tracking for pre-grasp planning on mobile robots
Abstract: The ability to track the 6D pose distribution of an object when a mobile manipulator robot is still approaching the object can enable the robot to pre-plan grasps that combine base and arm motion. However, tracking a 6D object pose distribution from a distance can be challenging due to the limited view of the robot camera. In this work, we present a framework that fuses observations from external stationary cameras with a moving robot camera and sequentially tracks it in time to enable 6D object pose distribution tracking from a distance. We model the object pose posterior as a multi-modal distribution which results in a better performance against uncertainties introduced by large camera-object distance, occlusions and object geometry.
Multi-view object pose distribution tracking for pre-grasp planning on mobile robots
Abstract: The ability to track the 6D pose distribution of an object when a mobile manipulator robot is still approaching the object can enable the robot to pre-plan grasps that combine base and arm motion. However, tracking a 6D object pose distribution from a distance can be challenging due to the limited view of the robot camera. In this work, we present a framework that fuses observations from external stationary cameras with a moving robot camera and sequentially tracks it in time to enable 6D object pose distribution tracking from a distance. We model the object pose posterior as a multi-modal distribution which results in a better performance against uncertainties introduced by large camera-object distance, occlusions and object geometry.
Towards smooth & efficient manipulation on mobile robots using temporal integration
Abstract: Many of the tasks performed by mobile service robots today require navigation (approaching objects) and manipulation (interacting with the objects). Navigation & manipulation are often solved sequentially as separate tasks. Ideally, the robot can use the approaching motion for the manipulation task to improve the object’s perception as well as to pre-plan the manipulation for efficient execution. This PhD project is investigating how approaching motion can be used to optimize perception and planning to enable robust and efficient manipulation on mobile robots.
teaching
Robots and Autonomous Systems (RAS) - Fall 2017
Course incharge: Prof. Dr. Erwin Prassler
Robots and Autonomous Systems (RAS) - Spring 2019
Course incharge: Prof. Dr. Erwin Prassler
Deep Neural Networks (DNN) - Fall 2021
Course incharge: Assc. Prof. Dr. Anders Buch
Statistical Machine Learning (SML) - Spring 2022
Course incharge: Prof. Dr. Norbert Kruger
Statistical Machine Learning (SML) - Spring 2023
Course incharge: Prof. Dr. Norbert Kruger