Posts by Collection
publications
research
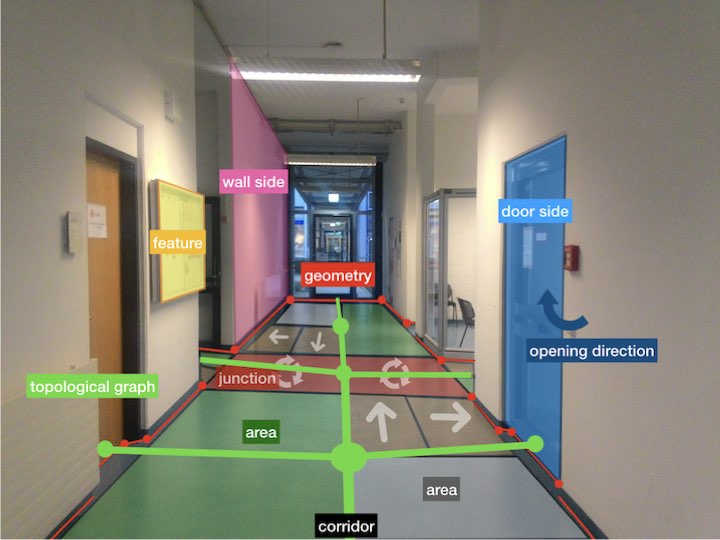
Semantic world modelling and indoor navigation using OpenStreetMap
We explored how digital navigation maps such as OpenStreetMap can be used for indoor robot navigation
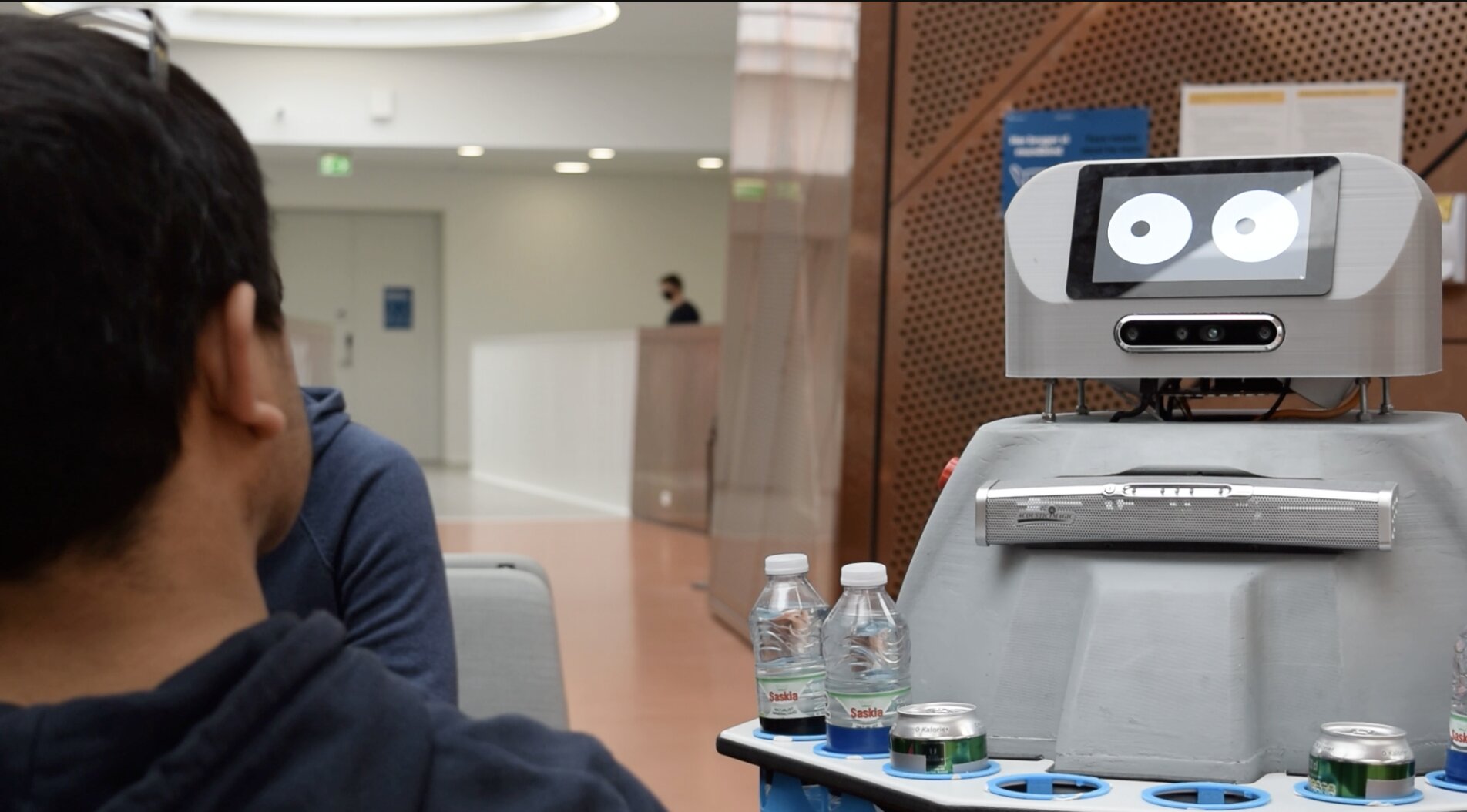
An interactive drink serving robot
We designed and implemented an interactive service robot capable of seamless deployment in real-world environments, coupled with high social acceptance.
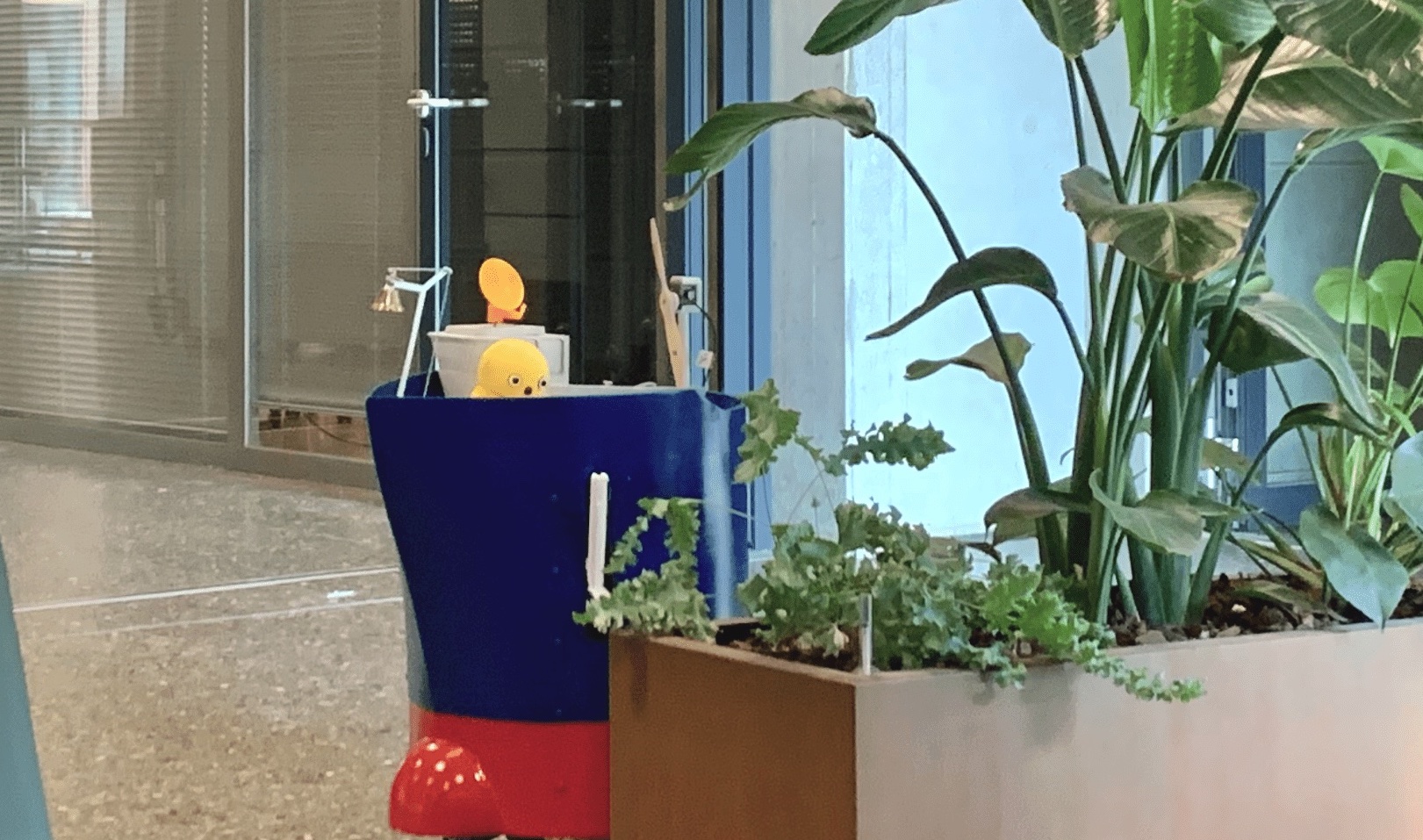
Distributed agency in HRI
We explored the use of distributed agency on mobile care robots using a prototype of the Plant Watering Robot
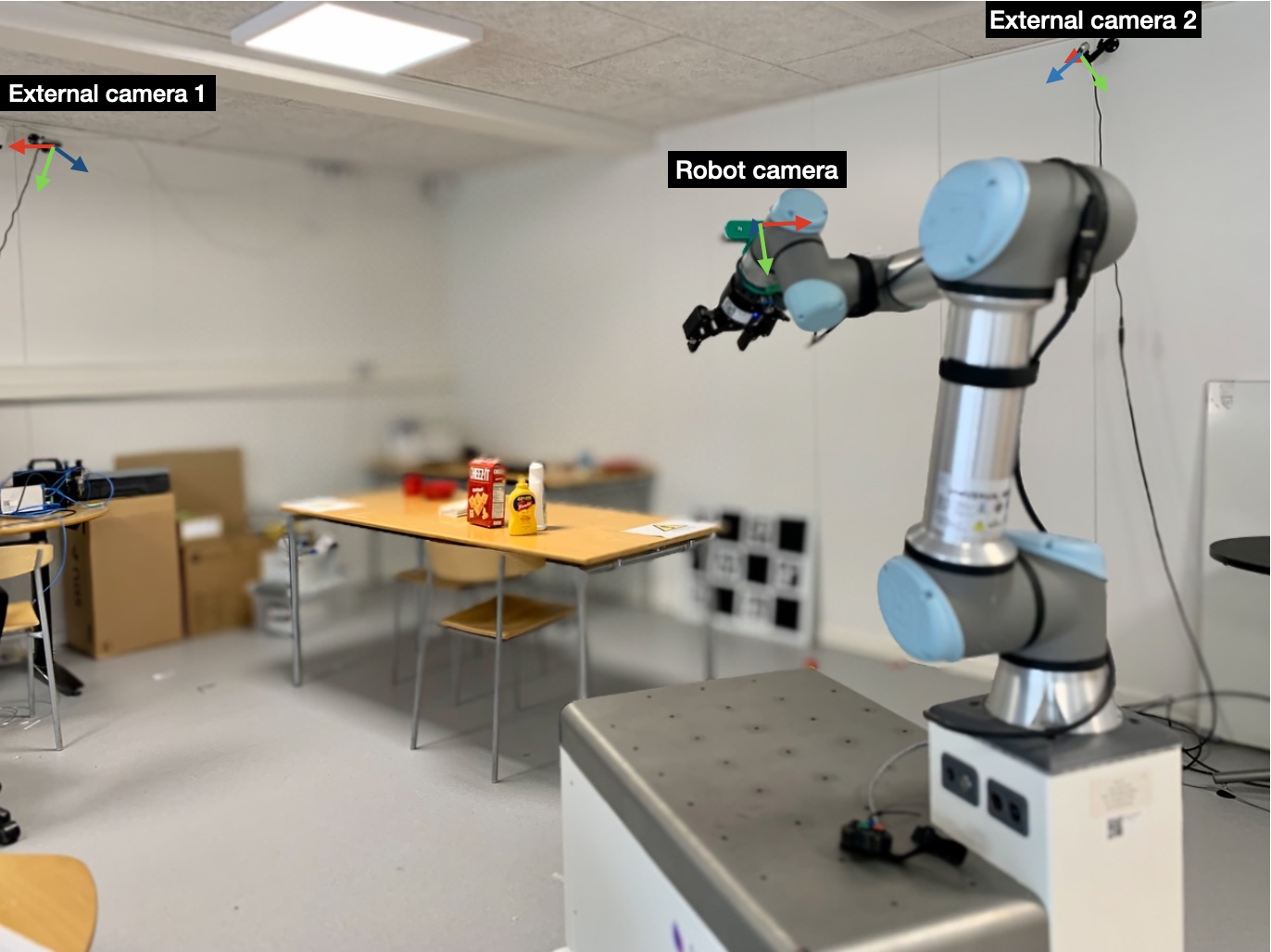
Multi-view object pose distribution tracking
We developed multi-view object pose distribution tracking framework for pre-grasp planniong on mobile robots
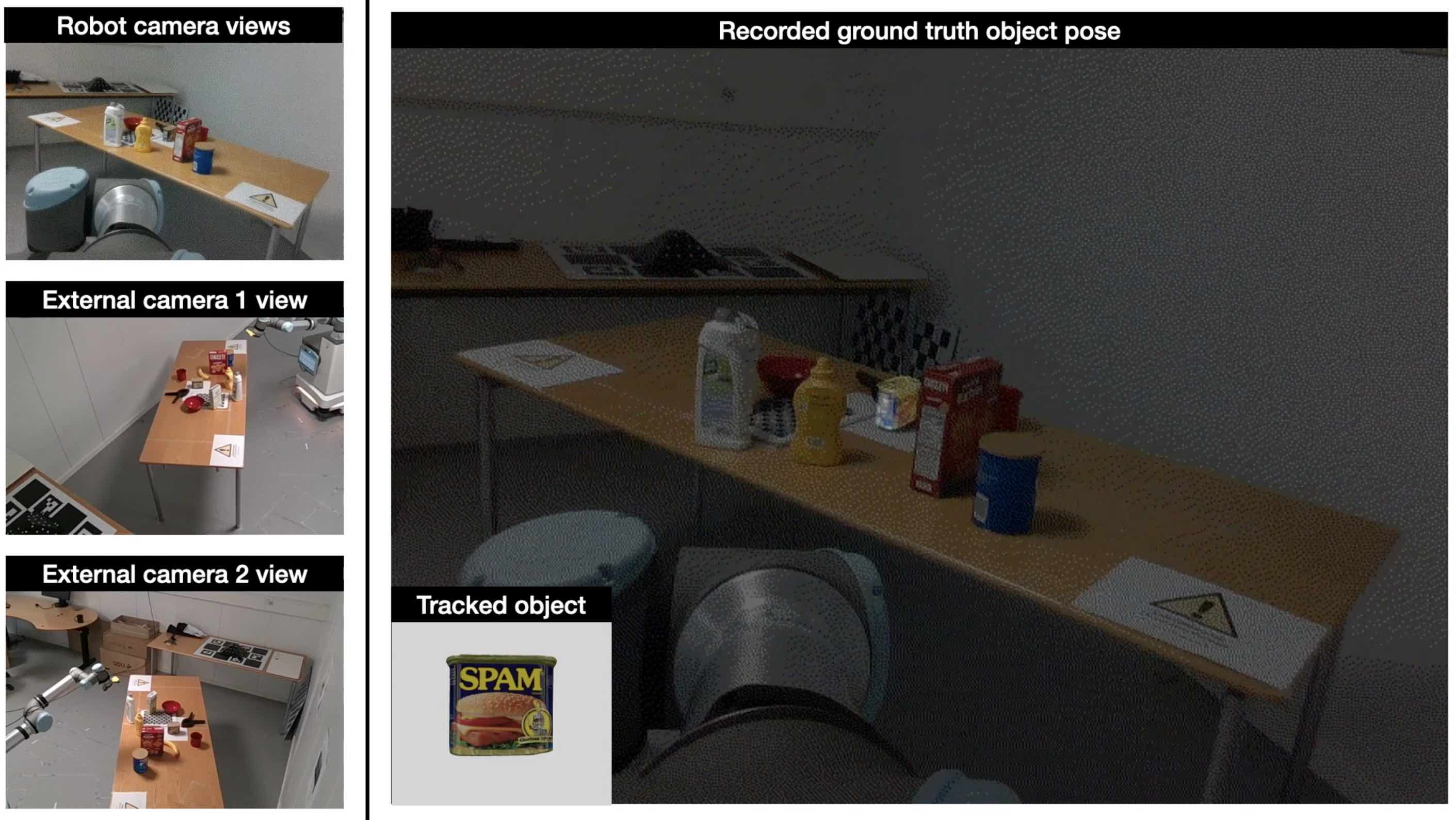
Multi-view YCB object pose tracking dataset for Mobile Manipulation
We released a Multi-view YCB object pose tracking dataset for Mobile Manipulation (MY-MM) with views of the objects from the robot's eye-in-hand and external cameras in the environment.
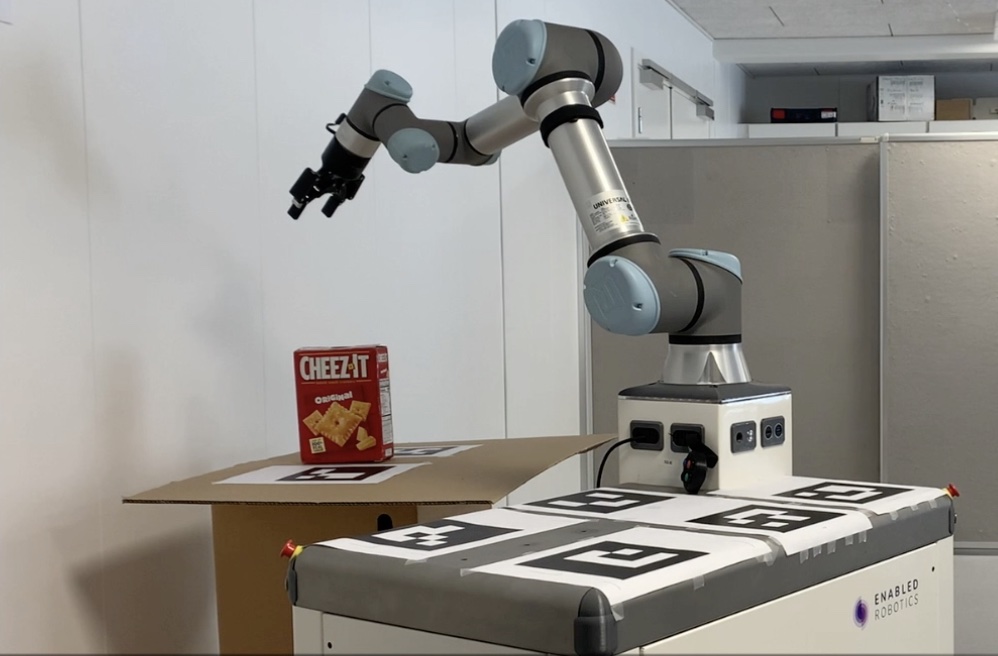
Pre-grasp approaching on mobile robots
In this research, we explored the use of a pre-active approach to determine a suitable base pose and pre-grasp manipulator configuration for grasping on mobile robots.
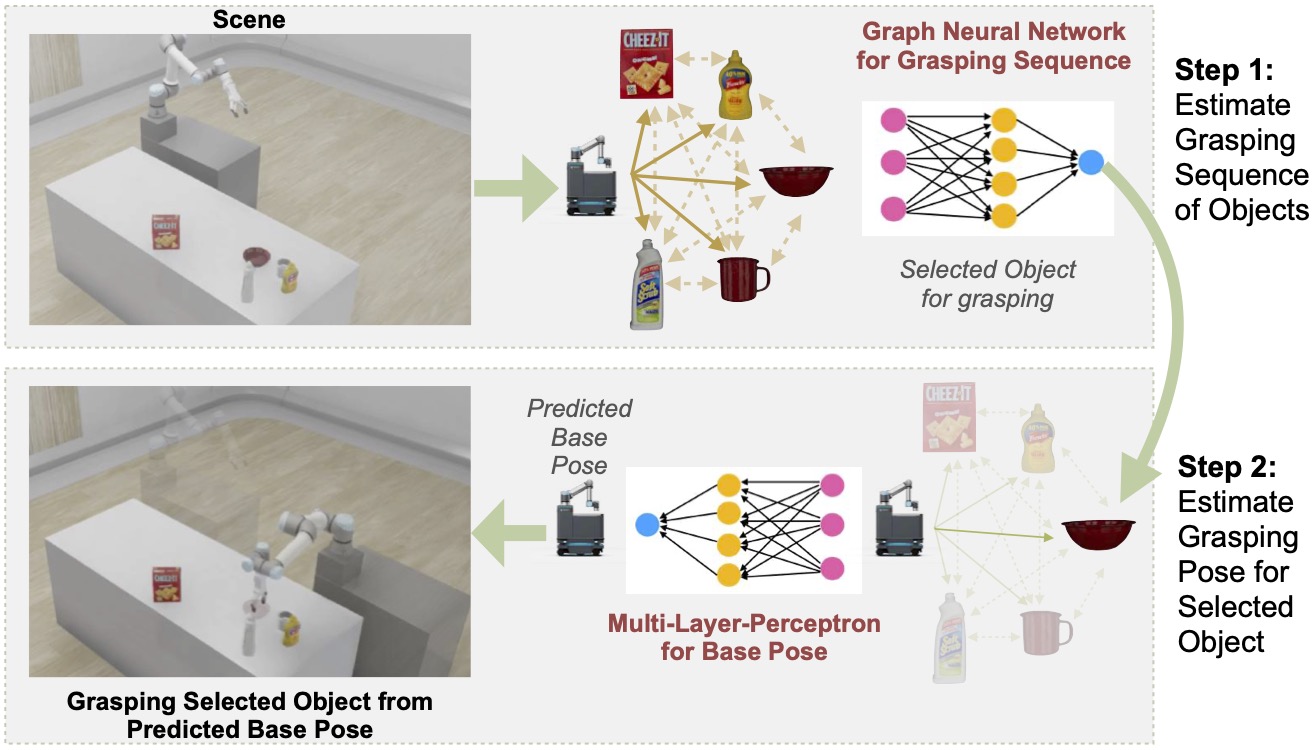
BaSeNET: A Learning-based Mobile Manipulator Base Pose Sequence Planning for Pickup Tasks
We present BaSeNET: a learning-based approach to plan the sequence of base poses for grasping objects on mobile robots.
talks
Semantic mapping extension for OpenStreetMap applied to indoor robot navigation
Abstract: In this work a graph-based, semantic mapping approach for indoor robotics applications is presented, which is extending OpenStreetMap (OSM) with robotic-specific, semantic, topological, and geometrical information. Models are introduced for basic indoor structures such as walls, doors, corridors, elevators, etc. The architectural principles support composition with additional domain and application-specific knowledge. As an example, a model for an area is introduced, and it is explained how this can be used in navigation. A key advantage of the proposed graph-based map representation is that it allows exploiting the hierarchical structure of the graphs. Finally, the compatibility of the approach with existing, grid-based motion planning algorithms is shown.
Multi-modal Proactive Approaching of Humans for Human-Robot Cooperative Tasks
Abstract: In this paper, we present a method for proactive approaching of humans for human-robot cooperative tasks such as a robot serving beverages to people. The proposed method can deal robustly with the uncertainties in the robot’s perception while also ensuring socially acceptable behavior. We use multiple modalities in the form of the robot’s motion, body orientation, speech and gaze to proactively approach humans. Further, we present a behavior tree based control architecture to efficiently integrate these different modalities. The proposed method was successfully integrated and tested on a beverage serving robot. We present the findings of our experiments and discuss possible extensions to address limitations.
Multi-view object pose distribution tracking for pre-grasp planning on mobile robots
Abstract: The ability to track the 6D pose distribution of an object when a mobile manipulator robot is still approaching the object can enable the robot to pre-plan grasps that combine base and arm motion. However, tracking a 6D object pose distribution from a distance can be challenging due to the limited view of the robot camera. In this work, we present a framework that fuses observations from external stationary cameras with a moving robot camera and sequentially tracks it in time to enable 6D object pose distribution tracking from a distance. We model the object pose posterior as a multi-modal distribution which results in a better performance against uncertainties introduced by large camera-object distance, occlusions and object geometry.
Multi-view object pose distribution tracking for pre-grasp planning on mobile robots
Abstract: The ability to track the 6D pose distribution of an object when a mobile manipulator robot is still approaching the object can enable the robot to pre-plan grasps that combine base and arm motion. However, tracking a 6D object pose distribution from a distance can be challenging due to the limited view of the robot camera. In this work, we present a framework that fuses observations from external stationary cameras with a moving robot camera and sequentially tracks it in time to enable 6D object pose distribution tracking from a distance. We model the object pose posterior as a multi-modal distribution which results in a better performance against uncertainties introduced by large camera-object distance, occlusions and object geometry.
Multi-view object pose distribution tracking for pre-grasp planning on mobile robots
Abstract: The ability to track the 6D pose distribution of an object when a mobile manipulator robot is still approaching the object can enable the robot to pre-plan grasps that combine base and arm motion. However, tracking a 6D object pose distribution from a distance can be challenging due to the limited view of the robot camera. In this work, we present a framework that fuses observations from external stationary cameras with a moving robot camera and sequentially tracks it in time to enable 6D object pose distribution tracking from a distance. We model the object pose posterior as a multi-modal distribution which results in a better performance against uncertainties introduced by large camera-object distance, occlusions and object geometry.
Multi-view object pose distribution tracking for pre-grasp planning on mobile robots
Abstract: The ability to track the 6D pose distribution of an object when a mobile manipulator robot is still approaching the object can enable the robot to pre-plan grasps that combine base and arm motion. However, tracking a 6D object pose distribution from a distance can be challenging due to the limited view of the robot camera. In this work, we present a framework that fuses observations from external stationary cameras with a moving robot camera and sequentially tracks it in time to enable 6D object pose distribution tracking from a distance. We model the object pose posterior as a multi-modal distribution which results in a better performance against uncertainties introduced by large camera-object distance, occlusions and object geometry.
Pre-grasp planning for time-efficient and robust mobile manipulation
Abstract: In Mobile Manipulation (MM), navigation and manipulation actions are commonly addressed sequentially. The time efficiency of MM can be improved by simultaneously planning pre-grasp manipulation actions while the robot performs the navigation actions. However, planning pre-grasp manipulation actions requires accurate 6D object poses, which are usually available only when the robot is close to and has a clear view of the objects. Further, pre-grasp planning with uncertain poses can lead to failures. This thesis explores how to provide reliable object poses for pre-grasp planning along with their associated uncertainties while the robot is still approaching the objects for grasping, and how to use these pose estimates to plan pre-grasp actions and make informed decisions to enhance the time efficiency and robustness of mobile manipulation.
Pre-grasp approaching on mobile robots: a pre-active layered approach
Abstract: In Mobile Manipulation (MM), navigation and manipulation are generally solved as subsequent disjoint tasks. Combined optimization of navigation and manipulation costs can improve the time efficiency of MM. However, this is challenging as precise object pose estimates, which are necessary for such combined optimization, are often not available until the later stages of MM. Moreover, optimizing navigation and manipulation costs with conventional planning methods using uncertain object pose estimates can lead to failures and hence requires re-planning. Hence, in the presence of object pose uncertainty, pre-active approaches are preferred.
BaSeNET: A Learning-based Mobile Manipulator Base Pose Sequence Planning for Pickup Tasks
Addressed Problem: In many applications, a mobile manipulator robot is required to grasp a set of objects distributed in space. This may not be feasible from a single base pose and the robot must plan the sequence of base poses for grasping all objects, minimizing the total navigation and grasping time. This is a Combinatorial Optimization problem that can be solved using exact methods, which provide optimal solutions but are computationally expensive, or approximate methods, which offer computationally efficient but sub-optimal solutions. Recent studies have shown that learning-based methods can solve Combinatorial Optimization problems, providing nearoptimal and computationally efficient solutions.
teaching
Robots and Autonomous Systems (RAS) - Fall 2017
Course incharge: Prof. Dr. Erwin Prassler
Robots and Autonomous Systems (RAS) - Spring 2019
Course incharge: Prof. Dr. Erwin Prassler
Deep Neural Networks (DNN) - Fall 2021
Course incharge: Assc. Prof. Dr. Anders Buch
Statistical Machine Learning (SML) - Spring 2022
Course incharge: Prof. Dr. Norbert Kruger
Statistical Machine Learning (SML) - Spring 2023
Course incharge: Prof. Dr. Norbert Kruger